Table of Contents
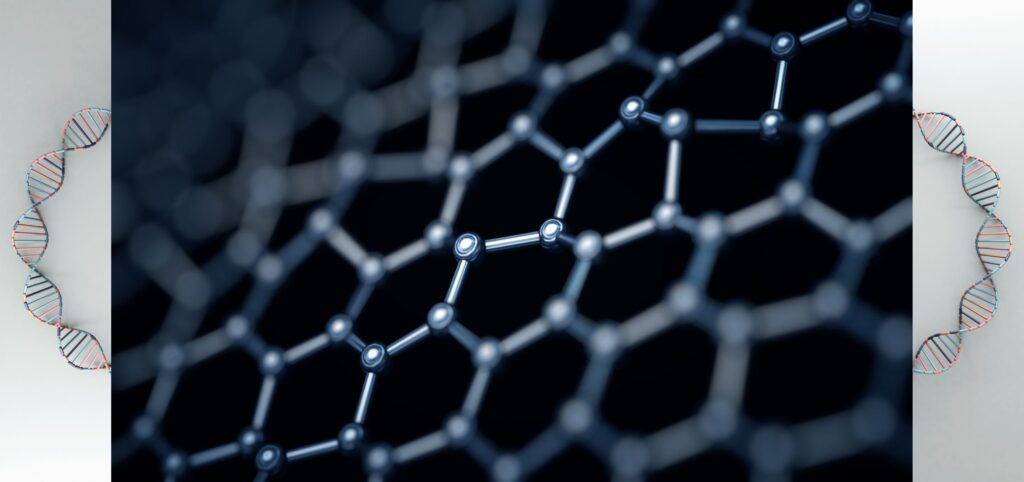
Introduction
AI in protein folding is revolutionizing our understanding of this fundamental biological process, where a protein chain acquires its functional three-dimensional structure. Understanding how proteins fold is crucial for various scientific and medical fields, including drug discovery and disease treatment. Despite its importance, the protein folding problem has been one of the most challenging puzzles in molecular biology for decades. However, recent advances in artificial intelligence (AI) have begun to transform our approach to this complex issue. This article explores the role of AI in protein folding in solving mysteries, detailing its implications, breakthroughs, and future prospects.
The Importance of Protein Folding
Proteins are essential macromolecules in all living organisms, performing a vast array of functions necessary for life. They are involved in catalyzing biochemical reactions, providing structural support, transporting molecules, and regulating cellular processes. The specific function of a protein is determined by its three-dimensional structure, which is formed through a process called protein folding. Misfolded proteins can lead to various diseases, including Alzheimer’s, Parkinson’s, and cystic fibrosis. Therefore, understanding protein folding is not only critical for basic biology but also for developing therapeutic interventions.
The Challenge of Protein Folding
The process by which a protein folds into its functional shape is governed by the sequence of amino acids in the protein chain. Predicting this three-dimensional structure from the amino acid sequence, known as the protein folding problem, has been a significant scientific challenge. Traditional experimental methods, such as X-ray crystallography and nuclear magnetic resonance (NMR) spectroscopy, are time-consuming and expensive. Computational approaches, including molecular dynamics simulations, have also struggled due to the immense complexity and size of the problem.
Breakthroughs – AI in Protein Folding
DeepMind’s AlphaFold
One of the most notable breakthroughs in protein folding has come from DeepMind, a subsidiary of Alphabet Inc., with their AI system called AlphaFold. In 2020, AlphaFold demonstrated unprecedented accuracy in predicting protein structures, winning the Critical Assessment of protein Structure Prediction (CASP) competition. AlphaFold’s success marked a significant milestone, showing that AI could outperform traditional methods in solving the protein folding problem.
How AlphaFold Works
AlphaFold uses a deep learning approach, training neural networks on large datasets of known protein structures. The system incorporates both sequence information and evolutionary data to predict the distances between pairs of amino acids and the angles of chemical bonds. By integrating these predictions, AlphaFold constructs highly accurate three-dimensional models of proteins. The accuracy of AlphaFold’s predictions has been validated against experimental data, making it a powerful tool for researchers.
Implications for Biomedical Research
The ability of AI to predict protein structures accurately has far-reaching implications for biomedical research. It accelerates the process of understanding protein functions and interactions, facilitating drug discovery and the development of new therapies. For instance, knowing the precise structure of a protein can help identify potential binding sites for drugs, leading to the design of more effective and targeted treatments. Additionally, AI-driven protein folding predictions can aid in understanding diseases caused by protein misfolding, providing insights into potential interventions.
AI’s Expanding Role in Protein Folding
Beyond AlphaFold: Other AI Models
While AlphaFold has garnered much attention, other AI models and approaches are also contributing to advancements in protein folding. For example, RoseTTAFold, developed by researchers at the University of Washington, has shown similar success in predicting protein structures. These models use different architectures and training methodologies, enriching the field with diverse perspectives and techniques.
AI and Protein-Protein Interactions
Understanding protein-protein interactions is another critical aspect of molecular biology. AI models are increasingly being used to predict how proteins interact with each other, which is essential for understanding cellular processes and developing new therapies. Predicting these interactions involves complex calculations and vast amounts of data, making AI an ideal tool for this task. Improved predictions of protein-protein interactions can lead to better understanding of cellular mechanisms and the identification of novel drug targets.
AI and Protein Design
AI is also playing a crucial role in the field of protein design, where researchers aim to create new proteins with specific functions. By predicting how changes in amino acid sequences affect protein folding and stability, AI can guide the design of proteins with desired properties. This capability has significant implications for biotechnology and medicine, enabling the development of novel enzymes, therapeutic proteins, and biomaterials.
The Future of AI in Protein Folding
Integrating AI with Experimental Methods
While AI has made remarkable strides in protein folding, integrating AI predictions with experimental methods will be essential for further advancements. AI models can guide and refine experimental studies, reducing the time and cost associated with traditional techniques. Conversely, experimental data can improve AI models by providing additional training examples and validating predictions. This synergy between AI and experimental approaches promises to accelerate progress in understanding protein folding and its applications.
Challenges and Limitations
Despite its successes, AI in protein folding is not without challenges and limitations. One significant challenge is the availability and quality of training data. AI models require large datasets of accurately determined protein structures, which can be limited for certain types of proteins or organisms. Additionally, AI predictions may sometimes lack the precision needed for specific applications, necessitating further refinement and validation. Addressing these challenges will require ongoing collaboration between computational scientists, biologists, and experimentalists.
Ethical Considerations
As with any technological advancement, the use of AI in protein folding raises ethical considerations. Ensuring transparency and reproducibility of AI predictions is crucial for scientific integrity. Moreover, the potential for AI to be used in bioweapon development or other malicious applications underscores the need for responsible research and regulation. Engaging in open dialogue and establishing ethical guidelines will be vital to harness the benefits of AI while mitigating its risks.
Conclusion
The application of AI in protein folding represents a revolutionary advancement in molecular biology. Systems like AlphaFold have demonstrated the potential of AI to solve complex scientific problems, providing accurate predictions of protein structures and opening new avenues for research and innovation. As AI continues to evolve, its role in protein folding and related fields is expected to expand, driving progress in biomedical research, drug discovery, and biotechnology. By integrating AI with experimental methods and addressing ethical considerations, the scientific community can unlock the full potential of AI in understanding and manipulating the fundamental building blocks of life.
References
https://www.nature.com/articles/s41586-019-1923-7
https://www.nature.com/articles/s41586-021-03819-2
https://www.ncbi.nlm.nih.gov/pmc/articles/PMC7612213
https://www.ncbi.nlm.nih.gov/pmc/articles/PMC10968151
https://www.ncbi.nlm.nih.gov/pmc/articles/PMC9580946
https://www.ncbi.nlm.nih.gov/pmc/articles/PMC9442638
Videohttps://youtube.com/shorts/FiP5zi1WNZc?feature=share