Table of Contents
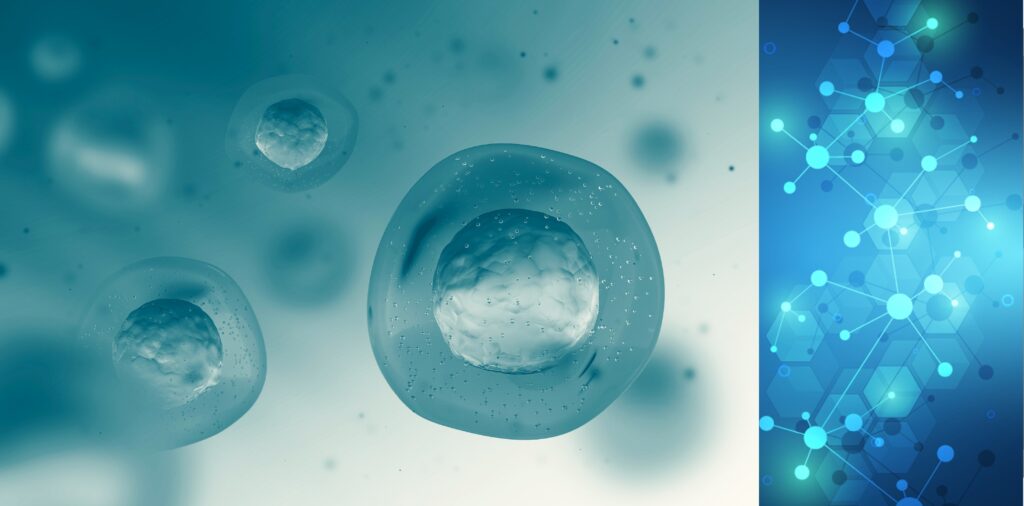
Discover how AI is transforming spheroid research, offering new insights into cancer, tissue engineering, and drug discovery. Learn how machine learning enhances image analysis, predictive modeling, and personalized medicine, paving the way for groundbreaking advancements in biomedical science. Stay tuned to understand the future of AI-driven spheroids and its impact on healthcare
Introduction
In the realm of biomedical research, spheroids represent a promising frontier, offering more accurate models of human tissue than traditional 2D cell cultures. As we push the boundaries of this exciting field, Artificial Intelligence (AI) is playing a crucial role in driving innovations. This article delves into the ways AI is revolutionizing spheroids research, enhancing our understanding, and expediting breakthroughs in medical science.
What Are Spheroids?
Spheroids are three-dimensional cell culture systems that closely mimic the natural environment of tissues in the body. They offer significant advantages over 2D cultures, including better cell-cell and cell-matrix interactions, more accurate responses to stimuli, and the ability to replicate complex biological processes. This makes them invaluable for studying cancer, tissue regeneration, drug screening, and personalized medicine.
Benefits of Spheroids
- Enhanced Cell Interactions: Spheroids provide a more realistic environment for cells to interact with each other and their surroundings, mirroring the natural conditions found in human tissues.
- Improved Drug Testing: By using spheroids, researchers can obtain more accurate data on how drugs affect cells, leading to better predictions of a drug’s efficacy and safety.
- Reduced Animal Testing: Spheroids can reduce the need for animal testing by providing a more relevant human cell model for preliminary experiments.
Types of Spheroids
- Tumor Spheroids: Used primarily in cancer research to study tumor biology, drug resistance, and metastasis.
- Stem Cell Spheroids: Utilized in regenerative medicine and tissue engineering to explore stem cell differentiation and tissue formation.
- Hepatocyte Spheroids: Employed in liver research for drug metabolism and toxicity studies.
The Role of AI in Spheroids Research
1. Enhancing Image Analysis
AI has vastly improved the analysis of spheroid images. Traditional methods are time-consuming and prone to human error. Machine learning algorithms can process large volumes of image data rapidly and with high precision. These algorithms can identify and quantify spheroid characteristics such as size, shape, and viability, providing researchers with more accurate and comprehensive data.
Techniques in AI Image Analysis
- Deep Learning: Deep neural networks can analyze complex image data, identifying subtle features and patterns that are often missed by traditional methods.
- Computer Vision: This technology allows for the automated extraction of information from images, enhancing the speed and accuracy of spheroid analysis.
- High-Throughput Screening: AI can manage and analyze large datasets generated from high-throughput screening, significantly accelerating the research process.
2. Predictive Modeling
Predictive modeling powered by AI allows researchers to forecast the behavior of spheroids under various conditions. By analyzing vast datasets, AI can predict outcomes such as drug efficacy and toxicity, enabling more efficient and targeted research. This capability is particularly valuable in oncology, where understanding how cancer spheroids respond to treatments can inform personalized therapy plans.
Applications of Predictive Modeling
- Drug Response Prediction: AI models can predict how different drugs will affect spheroids, helping to identify the most promising candidates for further development.
- Toxicity Prediction: By forecasting potential toxic effects of new compounds, AI can prevent adverse outcomes and streamline the drug development process.
- Disease Progression Modeling: AI can simulate the progression of diseases within spheroids, providing insights into disease mechanisms and potential therapeutic targets.
3. Drug Discovery and Screening
AI accelerates the drug discovery process by streamlining the screening of compounds on spheroid models. Machine learning algorithms can analyze how different drugs interact with spheroids, identifying promising candidates more quickly than traditional methods. This not only speeds up the development of new therapies but also reduces costs and increases the likelihood of successful outcomes.
AI-Driven Drug Screening
- Virtual Screening: AI can perform virtual screening of thousands of compounds, predicting their interactions with spheroids and identifying the most promising candidates for experimental validation.
- Multi-Parametric Analysis: AI can analyze multiple parameters simultaneously, such as cell viability, proliferation, and apoptosis, providing a comprehensive assessment of drug effects.
- Optimization of Drug Combinations: AI can identify optimal combinations of drugs that work synergistically to enhance therapeutic efficacy and minimize side effects.
4. Automated Culturing Systems
Automation, driven by AI, is transforming the way spheroids are cultured. Automated systems can manage the complex process of spheroid formation, growth, and maintenance, ensuring consistency and reproducibility. This reduces variability and human error, leading to more reliable experimental results.
Features of Automated Culturing
- Robotic Handling: Robots can handle the delicate process of spheroid formation, reducing the risk of contamination and ensuring uniformity.
- Real-Time Monitoring: AI-driven systems can continuously monitor spheroid growth and health, making adjustments as needed to maintain optimal conditions.
- Scalability: Automated systems can scale up the production of spheroids, supporting large-scale experiments and high-throughput screening.
5. Personalized Medicine
AI’s ability to analyze individual patient data and predict how their cells will behave in spheroid models is paving the way for personalized medicine. By creating patient-specific spheroids, researchers can test how different treatments will affect an individual’s cells, leading to more tailored and effective therapies.
Personalized Approaches
- Patient-Derived Spheroids: Spheroids created from a patient’s own cells can be used to test the effectiveness of various treatments, ensuring the chosen therapy is likely to be effective for that specific patient.
- Genomic Data Integration: AI can integrate genomic data with spheroid models to identify genetic factors that influence drug response, allowing for more precise treatment strategies.
- Adaptive Treatment Plans: AI can continuously analyze treatment outcomes and adjust therapy plans in real-time, optimizing patient care.
Applications
Cancer Research
One of the most significant applications of AI in spheroid research is in cancer studies. AI algorithms can analyze the complex interactions within cancer spheroids, helping to unravel the mechanisms of tumor growth and metastasis. This knowledge is critical for developing targeted therapies and improving patient outcomes.
Advances in Cancer Research
- Tumor Microenvironment Analysis: AI can study the tumor microenvironment within spheroids, providing insights into how cancer cells interact with their surroundings and identifying potential therapeutic targets.
- Drug Resistance Mechanisms: AI can uncover mechanisms of drug resistance in cancer spheroids, guiding the development of strategies to overcome resistance and improve treatment efficacy.
- Precision Oncology: By integrating patient data with spheroid models, AI can support the development of personalized treatment plans, enhancing the precision and effectiveness of cancer therapies.
Tissue Engineering
In tissue engineering, AI aids in the design and optimization of spheroid-based scaffolds. By simulating different conditions and predicting the best approaches, AI helps researchers create more effective and functional tissue constructs. This has enormous implications for regenerative medicine and the development of artificial organs.
Innovations in Tissue Engineering
- Scaffold Design: AI can optimize the design of scaffolds used to support spheroid growth, ensuring the best conditions for tissue formation and function.
- Bioprinting: AI-driven bioprinting technologies can create complex tissue structures with high precision, advancing the field of regenerative medicine.
- Functional Integration: AI can guide the integration of different cell types within spheroids, ensuring the formation of functional tissues that closely mimic natural organs.
Drug Toxicity Testing
AI-powered spheroid models are increasingly used for drug toxicity testing. These models provide a more accurate representation of how drugs affect human tissues, leading to better safety profiles and reducing the need for animal testing. AI can quickly analyze the effects of various compounds, identifying potential toxicities early in the development process.
Enhancing Drug Safety
- Early Detection of Toxicity: AI can identify early signs of toxicity in spheroids, allowing for the timely removal of harmful compounds from the drug development pipeline.
- Mechanistic Insights: AI can provide insights into the mechanisms of drug toxicity, helping to identify safer and more effective compounds.
- Regulatory Compliance: AI-driven toxicity testing can support regulatory compliance by providing robust and reliable data on drug safety.
Challenges and Future Directions
Data Integration
One of the significant challenges in AI-driven spheroid research is the integration of diverse data types. Spheroids generate vast amounts of data from different sources, including imaging, genetic, and biochemical data. AI systems must be capable of integrating and interpreting this data holistically to provide meaningful insights.
Overcoming Data Integration Challenges
- Standardization: Developing standardized protocols for data collection and analysis can facilitate data integration and enhance the reliability of AI models.
- Interoperability: Ensuring that different data systems and formats can work together seamlessly is crucial for effective data integration.
- Advanced Analytics: Using advanced analytics and machine learning techniques can help to extract meaningful insights from complex and diverse datasets.
Ethical Considerations
As with any AI application in biomedical research, ethical considerations are paramount. Issues such as data privacy, the potential for bias in AI algorithms, and the need for transparency in AI-driven decision-making must be carefully managed to ensure ethical standards are maintained.
Addressing Ethical Concerns
- Data Privacy: Implementing robust data privacy measures can protect patient information and ensure compliance with regulatory requirements.
- Algorithmic Fairness: Ensuring that AI algorithms are free from bias and provide fair and equitable results is critical for maintaining ethical standards.
- Transparency: Providing transparency in AI-driven decision-making processes can build trust and ensure accountability in spheroid research.
Advancements in AI Algorithms
The future of spheroid research will be shaped by advancements in AI algorithms. Continued development in machine learning and deep learning techniques will enhance the accuracy and predictive power of AI models. These advancements will open new avenues for research and lead to even more groundbreaking discoveries.
Future Directions in AI
- Explainable AI: Developing AI models that can explain their decisions and predictions will enhance transparency and trust in AI-driven research.
- Transfer Learning: Using transfer learning techniques, where knowledge from one domain is applied to another, can accelerate advancements in spheroid research.
- Edge AI: Implementing AI on edge devices can enable real-time data analysis and decision-making, enhancing the efficiency and effectiveness of spheroid research.
Interdisciplinary Collaboration
The integration of AI in spheroid research requires collaboration across multiple disciplines, including biology, computer science, and engineering. Fostering these collaborations will be essential for overcoming technical challenges and maximizing the potential of AI-driven spheroid research.
Promoting Interdisciplinary Collaboration
- Cross-Disciplinary Training: Providing training opportunities for researchers from different disciplines can enhance their understanding of AI and its applications in spheroid research.
- Collaborative Research Projects: Encouraging collaborative research projects that bring together experts from various fields can drive innovation and accelerate advancements in spheroid research.
- Funding and Support: Providing funding and support for interdisciplinary research initiatives can foster collaboration and ensure the successful integration of AI in spheroid research.
Conclusion
AI is revolutionizing spheroid research, providing powerful tools to enhance our understanding of complex biological systems and accelerating the pace of medical breakthroughs. From improving image analysis to enabling personalized medicine, AI’s impact on spheroid research is profound and far-reaching. As we continue to advance in this field, the synergy between AI and spheroids will undoubtedly lead to significant strides in biomedical science.
References
https://www.ncbi.nlm.nih.gov/pmc/articles/PMC4026212
https://www.ncbi.nlm.nih.gov/pmc/articles/PMC10419178
https://www.sciencedirect.com/science/article/pii/S2949747723000398
https://www.ncbi.nlm.nih.gov/pmc/articles/PMC5787088
Video- Created using Video GPT by VEED
https://scifocos.com/wp-content/uploads/2024/07/Spheroid-reserach.mp4